Freshly published: Robust Latent Representations via Cross-Modal Translation and Alignment
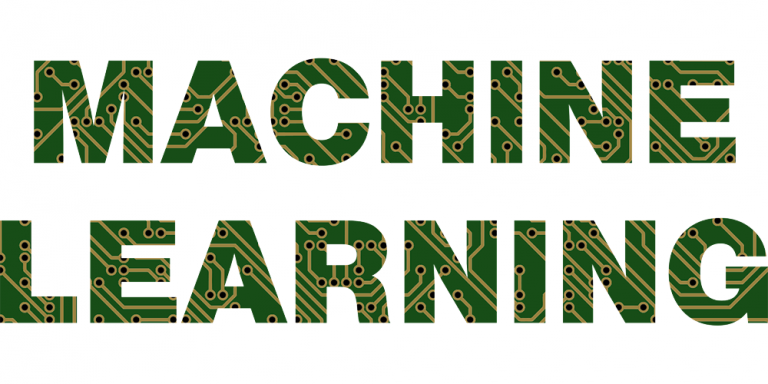
We are proud to mention that the FBK team just published one more scientific paper which is very relevant for MARVEL’s objectives. The paper’s title is: Robust Latent Representations via Cross-Modal Translation and Alignment and is available here. The authors are Vandana Rajan, Alessio Brutti, and Andrea Cavallaro and they will present the results in the IEEE International Conference on Acoustics, Speech, & Signal Processing (ICASSP). The conference will take place in Toronto on the 06th until the 11th of June, 2021.
Abstract of the paper
Multi-modal learning relates information across observation modalities of the same physical phenomenon to leverage complementary information. Most multi-modal machine learning methods require that all the modalities used for training are also available for testing. This is a limitation when signals from some modalities are unavailable or severely degraded. To address this limitation, we aim to improve the testing performance of uni-modal systems using multiple modalities during training only. The proposed multi-modal training framework uses cross-modal translation and correlation-based latent space alignment to improve the representations of a worse performing (or weaker) modality. The translation from the weaker to the better performing (or stronger) modality generates a multi-modal intermediate encoding that is representative of both modalities. This encoding is then correlated with the stronger modality representation in a shared latent space. We validate the proposed framework on the AVEC 2016 dataset (RECOLA) for continuous emotion recognition and show the effectiveness of the framework that achieves state-of-the-art (uni-modal) performance for weaker modalities.
For more direct updates from the MARVEL team follow us on LinkedIn and on Twitter. For regular updates including a collection of news and relevant information sign up on our Newsletter below.
Newsletter
Subscribe
Menu
- Home
- About
- Experimentation
- Knowledge Hub
- ContactResults
- News & Events
- Contact
Funding
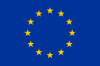
This project has received funding from the European Union’s Horizon 2020 Research and Innovation program under grant agreement No 957337. The website reflects only the view of the author(s) and the Commission is not responsible for any use that may be made of the information it contains.