Detailed info
Feedforward neural networks initialization based on discriminant learning
Authors: | Kateryna Chumachenko, Alexandros Iosifidis and Moncef Gabbouj |
Title: | Feedforward neural networks initialization based on discriminant learning |
Abstract: | In this paper, a novel data-driven method for weight initialization of Multilayer Perceptrons andConvolutional Neural Networks based on discriminant learning is proposed. The approach relaxes some of the limitations of competing data-driven methods, including unimodality assumptions, limitations on the architectures related to limited maximal dimensionalities of the corresponding projection spaces, as well as limitations related to high computational requirements due to the need of eigendecomposition on high-dimensional data. We also consider assumptions of the method on the data and propose a way to account for them in a form of a new normalization layer. The experiments on three large-scale image datasets show improved accuracy of the trained models compared to competing random-based and data-driven weight initialization methods, as well as better convergence properties in certain cases. |
Publication type: | Journal |
Title of the journal: | Neural Networks |
Year of Publication | 2021 |
Pages: | 220-229 |
Number, date or frequency of the Journal: | 146 |
Publisher: | Elsevier |
Url: | https://zenodo.org/record/5776561#.YbsgZlmxVPY |
DOI: | 10.1016/j.neunet.2021.11.020 |
Menu
- Home
- About
- Experimentation
- Knowledge Hub
- ContactResults
- News & Events
- Contact
Funding
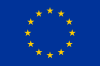
This project has received funding from the European Union’s Horizon 2020 Research and Innovation program under grant agreement No 957337. The website reflects only the view of the author(s) and the Commission is not responsible for any use that may be made of the information it contains.