Detailed info
Within-layer Diversity Reduces Generalization Gap
Authors | Firas Laakom, Jenni Raitoharju, Alexandros Iosifidis and Moncef Gabbouj |
Title | Within-layer Diversity Reduces Generalization Gap |
Abstract | Neural networks are composed of multiple layers arranged in a hierarchical structure jointly trained with a gradient-based optimization. At each optimization step, neurons at a given layer receive feedback from neurons belonging to higher layers of the hierarchy. In this paper, we propose to complement this traditional ’between-layer’ feed back with additional ’within-layer’ feedback to encourage diversity of the activations within the same layer. To this end, we measure the pairwise similarity between the outputs of the neurons and use it to model the layer’s overall diversity. By penalizing similarities and promoting diversity, we encourage each neuron to learn a distinctive representation and, thus, to enrich the data representation learned within the layer and to increase the total capacity of the model. We theoretically and empirically study how the within-layer activation diversity affects the generalization performance of a neural network and prove that increasing the diversity of hidden activations reduces the generalization gap. |
ISBN | Ν/Α |
Conference | ICML-21 Workshop on Information-Theoretic Methods for Rigorous, Responsible, and Reliable Machine Learning |
Date | 24/07/2021 |
Location | virtually |
Year of Publication, Publisher | 2021 |
Url | https://zenodo.org/record/5772760 |
DOI | 10.5281/zenodo.5772760 |
Menu
- Home
- About
- Experimentation
- Knowledge Hub
- ContactResults
- News & Events
- Contact
Funding
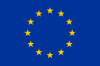
This project has received funding from the European Union’s Horizon 2020 Research and Innovation program under grant agreement No 957337. The website reflects only the view of the author(s) and the Commission is not responsible for any use that may be made of the information it contains.