Detailed info
Design Guidelines for Apache Kafka Driven Data Management and Distribution in Smart Cities
Authors | Theofanis P. Raptis, Claudio Cicconetti, Manolis Falelakis, Tassos Kanellos, Tomás Pariente Lobo |
Title | Design Guidelines for Apache Kafka Driven Data Management and Distribution in Smart Cities |
Abstract | Smart city management is going through a remark- able transition, in terms of quality and diversity of services provided to the end-users. The stakeholders that deliver pervasive applications are now able to address fundamental challenges in the big data value chain, from data acquisition, data analysis and processing, data storage and curation, and data visualisation in real scenarios. Industry 4.0 is pushing this trend forward, demanding for servitization of products and data, also for the smart cities sector where humans, sensors and devices are operating in strict collaboration. The data produced by the ubiquitous devices must be processed quickly to allow the implementation of reactive services such as situational awareness, video surveillance and geo-localization, while always ensuring the safety and privacy of involved citizens. This paper proposes a modular architecture to (i) leverage innovative technologies for data acquisition, management and distribution (such as Apache Kafka and Apache NiFi), (ii) develop a multi-layer engineering solution for revealing valuable and hidden societal knowledge in smart cities environment, and (iii) tackle the main issues in tasks involving complex data flows and provide general guidelines to solve them. We derived some guidelines from an experimental setting performed together with leading industrial technical departments to accomplish an efficient system for monitoring and servitization of smart city assets, with a scalable platform that confirms its usefulness in numerous smart city use cases with different needs. |
Conference | 2022 IEEE International Smart Cities Conference (ISC2) |
Date | 26/09/2022 |
Location | Paphos, Cyprus |
Year of Publication | 2022 |
Url | https://doi.org/10.5281/zenodo.10460843 |
DOI | 10.1109/ISC255366.2022.9922546 |
Menu
- Home
- About
- Experimentation
- Knowledge Hub
- ContactResults
- News & Events
- Contact
Funding
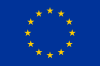
This project has received funding from the European Union’s Horizon 2020 Research and Innovation program under grant agreement No 957337. The website reflects only the view of the author(s) and the Commission is not responsible for any use that may be made of the information it contains.