Detailed Info
Dynamic hard pruning of Neural Networks at the edge of the internet
Authors: | Lorenzo Valerio, Franco Maria Nardini, Andrea Passarella, Raffaele Perego |
Title: | Dynamic hard pruning of Neural Networks at the edge of the internet |
Abstract: | Neural Networks (NN), although successfully applied to several Artificial Intelligence tasks, are often unnecessarily over-parametrized. In edge/fog computing, this might make their training prohibitive on resource-constrained devices, contrasting with the current trend of decentralizing intelligence from remote data centres to local constrained devices. Therefore, we investigate the problem of training effective NN models on constrained devices having a fixed, potentially small, memory budget. We target techniques that are both resource-efficient and performance effective while enabling significant network compression. Our Dynamic Hard Pruning (DynHP) technique incrementally prunes the network during training, identifying neurons that marginally contribute to the model accuracy. DynHP enables a tunable size reduction of the final neural network and reduces the NN memory occupancy during training. Freed memory is reused by a dynamic batch sizing approach to counterbalance the accuracy degradation caused by the hard pruning strategy, improving its convergence and effectiveness. We assess the performance of DynHP through reproducible experiments on three public datasets, comparing them against reference competitors. Results show that DynHP compresses a NN up to 10 times without significant performance drops (up to 3.5% additional error w.r.t. the competitors), reducing up to 80% the training memory occupancy. |
Publication type: | Journal |
Title of the journal: | Journal of Network and Computer Applications |
Year of Publication | 2022 |
Pages: | 1-11 |
Number, date or frequency of the Journal: | 200 |
Publisher: | Elsevier |
Url: | https://zenodo.org/record/6322721#.Yh9LKS8Rpqs |
DOI: | https://doi.org/10.1016/j.jnca.2021.103330 |
Menu
- Home
- About
- Experimentation
- Knowledge Hub
- ContactResults
- News & Events
- Contact
Funding
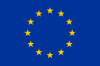
This project has received funding from the European Union’s Horizon 2020 Research and Innovation program under grant agreement No 957337. The website reflects only the view of the author(s) and the Commission is not responsible for any use that may be made of the information it contains.