Fairness in Machine Learning
A brief introduction
Machine learning has begun to invade all aspects of life, even those protected by anti-discrimination law. It is being used in hiring, credit, criminal justice, advertising, education, and more. What makes this situation particularly difficult, however, is that machine learning algorithms are more fluid and intangible. Part of designing complex machine learning algorithms is that they are difficult to interpret and regulate. Machine learning fairness is a growing field that seeks to cement the abstract principles of “fairness” in machine learning algorithms.
The research community has invested a great deal of effort in this area, as illustrated by the figure below.
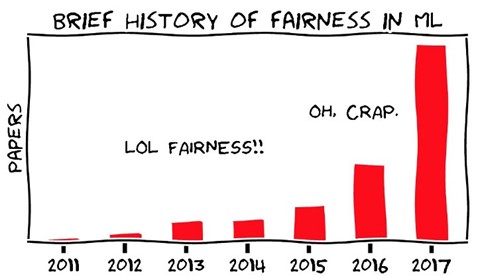
Motivations
The first question to ask is: Why do we care about fairness? The main motivation is that it is closely related to our own benefits. We are in an era where many things have become or are becoming automated by ML systems. Autonomous cars are around the corner and are estimated to be widely used within 5-10 years; employers are using ML to screen job applicants; US courts are using the COMPAS algorithm for recidivism prediction; Linked-in is using ML to rank interviewed candidates; etc. Machine learning systems are an integral part of our daily lives. They are becoming more widely used in the near future as more and more fields begin to integrate AI into their existing practices/products.
Artificial intelligence is good but it can be used incorrectly. Machine learning, the most widely used AI techniques, relies heavily on data. It is a common misconception that AI is absolutely objective. AI is only objective in the sense of learning what humans teach. Human-provided data can be highly biased. It was found in 2016 that COMPAS, the algorithm used for recidivism prediction, produces a much higher false positive rate for blacks than for whites (see figure below).

Causes
One would ask, “What causes bias in ML systems?” Essentially, the bias comes from the human bias that exists in the training dataset for historical reasons. Among the possible causes of bias in training datasets as classified by (Barocas et Selbst, 2016), we can find biased samples, an example of biased samples is a police station that has criminal records only in an area presenting a certain specific aspect (e.g., a noisy hood) and therefore the model will tend to learn that such a criterion is related to a high risk of crime.
Another cause is corrupted samples, where the collected data have been annotated by biased individuals, for example, a recruiter is annotating candidates qualification score while introducing bias, and thus the model will tend to pick the same recruiting pattern seen in the provided training samples.
One more cause, which is usually occurring, is the disparity of samples, where we can find that samples related to a minority group are less represented than others, and this will hinder the AI model from well modeling the minority group.
MARVEL and Fairness
Fortunately, MARVEL dedicates a specific work package for ethics, where fairness in Machine Learning is only part of it. Starting with fairness in Acoustic Scene Classification, active research and work is already ongoing and measures and practices are being taken. You can have a further read on our recent work here.
Menu
- Home
- About
- Experimentation
- Knowledge Hub
- ContactResults
- News & Events
- Contact
Funding
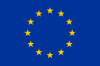
This project has received funding from the European Union’s Horizon 2020 Research and Innovation program under grant agreement No 957337. The website reflects only the view of the author(s) and the Commission is not responsible for any use that may be made of the information it contains.