Detailed Info
Large Deviations for Products of Non-Identically Distributed Network Matrices With Applications to Communication-Efficient Distributed Learning and Inference
Authors: | Petrović, Nemanja; Bajović, Dragana; Kar, Soummya; Jakovetić, Dušan; Sahu, Anit Kumar |
Title: | Large Deviations for Products of Non-Identically Distributed Network Matrices With Applications to Communication-Efficient Distributed Learning and Inference |
Abstract: | This paper studies products of independent but non-identically distributed random network matrices that arise as weight matrices in distributed consensus-type computation and inference procedures in peer-to-peer multi-agent networks. The non-identically distributed matrices studied in this paper model various application scenarios in which the agent communication network is time-varying, either naturally or engineered to achieve communication efficiency in computational procedures. First, under broad conditions on the statistics of the network matrix sequence, the product of the sequence is shown to converge almost surely to the consensus matrix and explicit large deviations rate of convergence are obtained. Specifically, given the admissible graph of interconnections modeling the base network topology, it is shown that the large deviations rate of consensus equals the minimum limiting value of the fluctuating graph cuts, where the edge costs are assigned through the current probabilities of the inter-agent communications. Secondly, an application of the above large deviations principle is studied in the context of distributed detection in time-varying networks with sequential observations. By adopting a consensus+innovations type distributed detection algorithm, as a by-product of this result, error exponents are obtained for the performance of distributed detection. It is shown that slow starts (slow increase) of inter-agent communication probabilities yield the same asymptotic error rate – and hence the same distributed detection performance, as if the communications were at their nominal levels from the beginning. As an important special case it is shown that when all the intermittent graph cuts have a link the probability of which increases to one, the performance of distributed detection is asymptotically optimal – i.e., equivalent to a centralized setup having access to all network data at all times. |
Publication type: | Journal |
Title of the journal: | |
Year of Publication | 2023 |
Number, date or frequency of the Journal: | Volume: 71 |
Publisher: | IEEE |
URL: | https://zenodo.org/records/10628857 |
DOI | 10.1109/TSP.2023.3263254 |
Menu
- Home
- About
- Experimentation
- Knowledge Hub
- ContactResults
- News & Events
- Contact
Funding
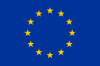
This project has received funding from the European Union’s Horizon 2020 Research and Innovation program under grant agreement No 957337. The website reflects only the view of the author(s) and the Commission is not responsible for any use that may be made of the information it contains.