Detailed info
Learning distinctive features helps, provably
Authors | Firas Laakom, Jenni Raitoharju, Alexandros Iosifidis and Moncef Gabbouj |
Title | Learning distinctive features helps, provably |
Abstract | We study the diversity of the features learned by a two-layer neural network trained with the least squares loss. We measure the diversity by the average L2-distance between the hidden-layer features and theoretically investigate how learning non-redundant distinct features affects the performance of the network. To do so, we derive novel generalization bounds depending on feature diversity based on Rademacher complexity for such networks. Our analysis proves that more distinct features at the network’s hidden units within the intermediate layer lead to better generalization. We also show how to extend our results to deeper networks and different losses. |
ISBN | TBA |
Conference | European Conference on Machine Learning and Principles and Practice of Knowledge Discovery in Databases (ECML PKDD 2023) |
Date | TBA |
Location | Turin, Italy |
Year of Publication | 2023 |
Url | https://zenodo.org/record/8086882 |
DOI |
Menu
- Home
- About
- Experimentation
- Knowledge Hub
- ContactResults
- News & Events
- Contact
Funding
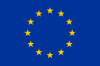
This project has received funding from the European Union’s Horizon 2020 Research and Innovation program under grant agreement No 957337. The website reflects only the view of the author(s) and the Commission is not responsible for any use that may be made of the information it contains.