Detailed info
Multi-Exit Vision Transformer for Dynamic Inference
Authors | Arian Bakhtiarnia, Qi Zhang, Alexandros Iosifidis |
Title | Multi-Exit Vision Transformer for Dynamic Inference |
Abstract | Deep neural networks can be converted to multi- exit architectures by inserting early exit branches after some of their intermediate layers. This allows their inference process to become dynamic, which is useful for time critical IoT applica- tions with stringent latency requirements, but with time-variant communication and computation resources. In particular, in edge computing systems and IoT networks where the exact computa- tion time budget is variable and not known beforehand. Vision Transformer is a recently proposed architecture which has since found many applications across various domains of computer vision. In this work, we propose seven different architectures for early exit branches that can be used for dynamic inference in Vision Transformer backbones. Through extensive experiments involving both classification and regression problems, we show that each one of our proposed architectures could prove useful in the trade-off between accuracy and speed. |
ISBN | TBD |
Conference | 32nd British Machine Vision Conference |
Date | 22-25/11/2021 |
Location | Virtual |
Year of Publication, Publisher | 2021 |
Url | https://zenodo.org/record/5604479 |
DOI | 10.5281/zenodo.5604479 |
Menu
- Home
- About
- Experimentation
- Knowledge Hub
- ContactResults
- News & Events
- Contact
Funding
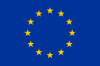
This project has received funding from the European Union’s Horizon 2020 Research and Innovation program under grant agreement No 957337. The website reflects only the view of the author(s) and the Commission is not responsible for any use that may be made of the information it contains.